In today’s rapidly evolving digital landscape, businesses increasingly rely on multi-cloud environments to enhance their operations, improve flexibility, and ensure data security. As the complexity of managing multiple cloud platforms grows, the role of machine learning (ML) in optimizing multi-cloud networks has become indispensable. This article delves into the ways machine learning is revolutionizing multi-cloud network optimization and how businesses can harness its power for seamless cloud management.
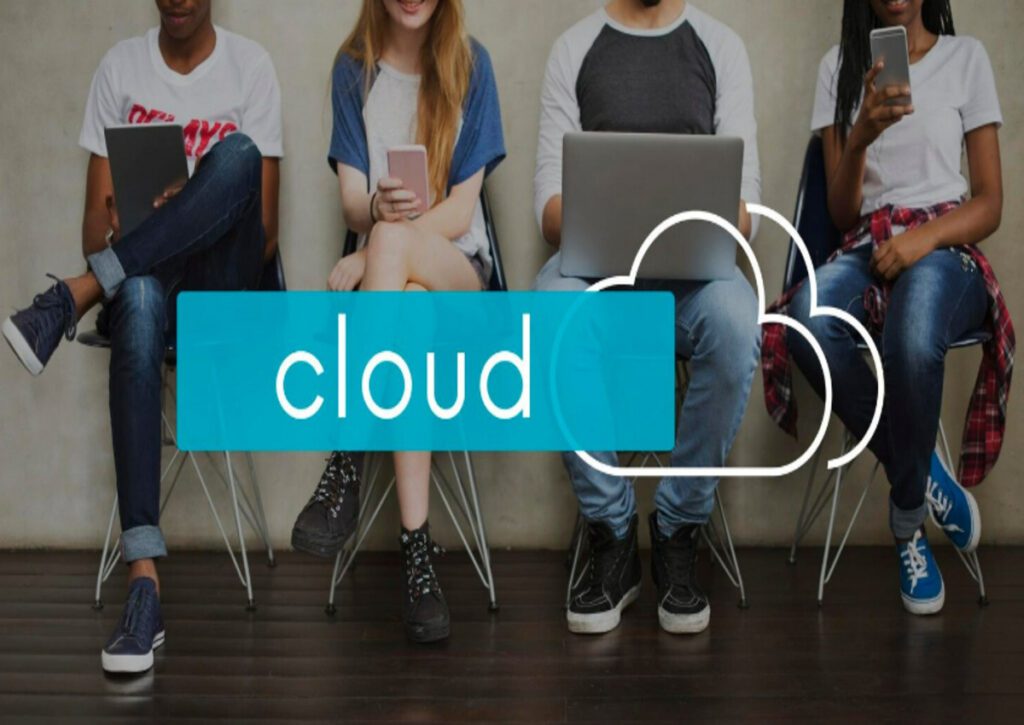
Understanding Multi-Cloud Networks
Multi-cloud networks involve the use of multiple cloud services from different providers, such as AWS, Google Cloud, and Microsoft Azure, to meet various business needs. This strategy allows organizations to avoid vendor lock-in, leverage the strengths of different cloud platforms, and ensure high availability and disaster recovery. However, managing such a diverse environment presents significant challenges, including network latency, security risks, and resource allocation inefficiencies.
How Machine Learning Enhances Multi-Cloud Network Optimization
Predictive Analytics for Proactive Management
Machine learning algorithms can analyze historical data to predict future network behavior. By identifying patterns and trends, ML models help in forecasting traffic loads, potential downtimes, and security threats. This proactive approach enables businesses to make informed decisions and take preemptive actions to maintain optimal network performance.
Automated Resource Allocation
One of the primary benefits of machine learning in multi-cloud environments is its ability to automate resource allocation. ML algorithms continuously monitor network usage and dynamically allocate resources to ensure efficient utilization. This reduces costs by preventing over-provisioning and improves performance by avoiding resource shortages.
Enhanced Security Measures
Security is a critical concern in multi-cloud networks. Machine learning enhances security by detecting anomalies and potential threats in real-time. ML models can differentiate between normal and suspicious activities, enabling rapid response to mitigate risks. This level of vigilance is crucial for protecting sensitive data across multiple cloud platforms.
Optimized Network Traffic Management
Machine learning algorithms can optimize network traffic by analyzing data packets and routing them through the most efficient paths. This reduces latency and improves the overall user experience. Additionally, ML can adapt to changing network conditions, ensuring consistent performance even during peak usage periods.
Cost Management and Optimization
Managing costs in a multi-cloud environment can be complex. Machine learning provides insights into spending patterns and identifies opportunities for cost savings. By optimizing resource usage and eliminating unnecessary expenses, businesses can achieve significant cost reductions without compromising on performance.
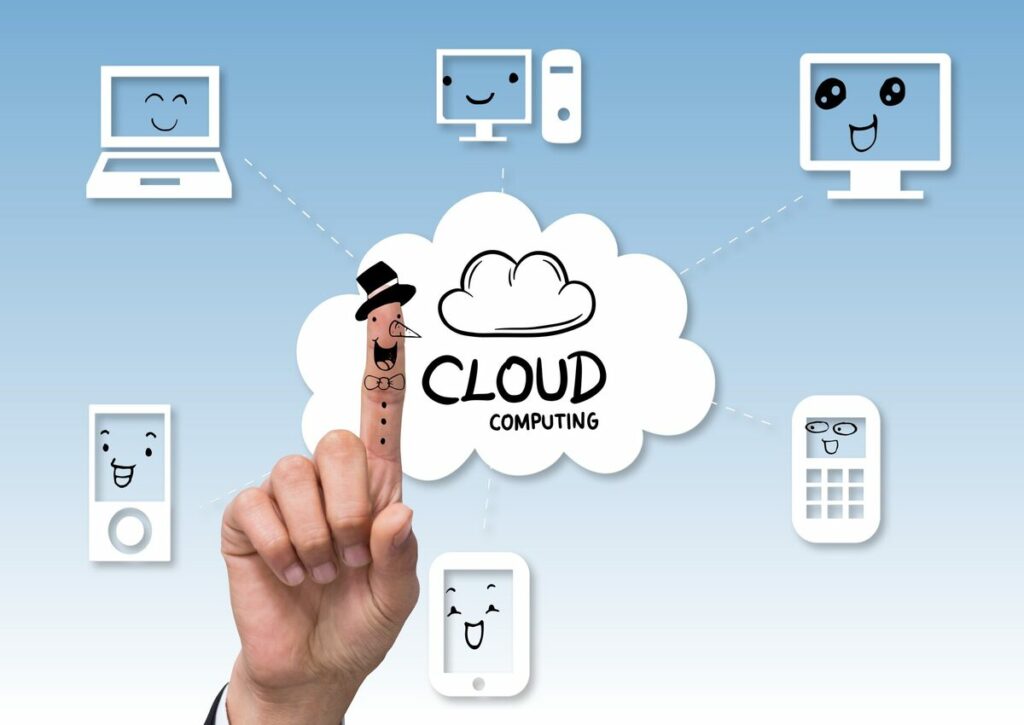
The Advantages of a Multi-Cloud ML Strategy
Implementing a multi-cloud machine learning strategy offers several advantages:
- Vendor Flexibility: Businesses are not tied to a single cloud provider, allowing them to choose the best services from different vendors to meet specific needs.
- Resilience and Reliability: Utilizing multiple cloud providers enhances system resilience. If one provider experiences an outage, operations can continue seamlessly on another platform.
- Scalability: A multi-cloud approach allows businesses to scale their operations more flexibly, taking advantage of the scalability features of different cloud providers.
- Innovation and Agility: Access to the latest technologies and innovations from various providers ensures that businesses can stay ahead of the competition and rapidly adapt to changing market demands.
How the Multi-Cloud Provides Extra Flexibility
The multi-cloud approach significantly enhances flexibility in several ways:
- Tailored Solutions: Businesses can select the most appropriate services from each cloud provider, creating a customized solution that best fits their requirements.
- Geographic Distribution: Utilizing multiple cloud providers allows for better geographic distribution of resources, reducing latency and improving access speed for global users.
- Avoidance of Vendor Lock-In: A multi-cloud strategy prevents dependency on a single vendor, giving businesses the freedom to switch providers or use multiple services without disruption.
- Regulatory Compliance: Different cloud providers may offer specific compliance certifications, enabling businesses to meet regulatory requirements more effectively.
The Challenges of a Multi-Cloud ML Strategy
While the benefits of a multi-cloud ML strategy are substantial, there are also challenges to consider:
- Complex Management: Coordinating multiple cloud environments can be complex, requiring advanced management tools and skilled personnel.
- Integration Issues: Ensuring seamless integration and interoperability between different cloud services can be challenging, necessitating robust integration solutions.
- Security Concerns: Managing security across multiple platforms can be difficult, requiring comprehensive security policies and practices to protect data.
- Cost Management: Keeping track of costs across various cloud providers can be challenging, necessitating detailed cost management strategies to avoid overspending.
Practical Applications of Machine Learning in Multi-Cloud Networks
- Load Balancing: Machine learning algorithms can distribute workloads evenly across multiple cloud platforms, preventing any single server from becoming a bottleneck. This ensures high availability and reliability of applications.
- Dynamic Scaling: ML models can predict traffic spikes and automatically scale resources up or down based on demand. This capability is particularly beneficial for e-commerce platforms and other businesses with fluctuating traffic patterns.
- Performance Monitoring: Continuous monitoring of network performance using machine learning helps in identifying and resolving issues promptly. This ensures that applications run smoothly and meet user expectations.
- Security Automation: Machine learning can automate security protocols such as intrusion detection and response, making it easier to manage security across diverse cloud environments.
- Data Analysis and Insights: Machine learning provides valuable insights by analyzing large volumes of data from multiple cloud sources. These insights can guide strategic decisions, such as which cloud services to prioritize or how to optimize resource distribution for better performance.
- Anomaly Detection: Machine learning models can detect unusual patterns that might indicate system malfunctions or security breaches. Early detection allows for quick intervention, minimizing potential damage and downtime.
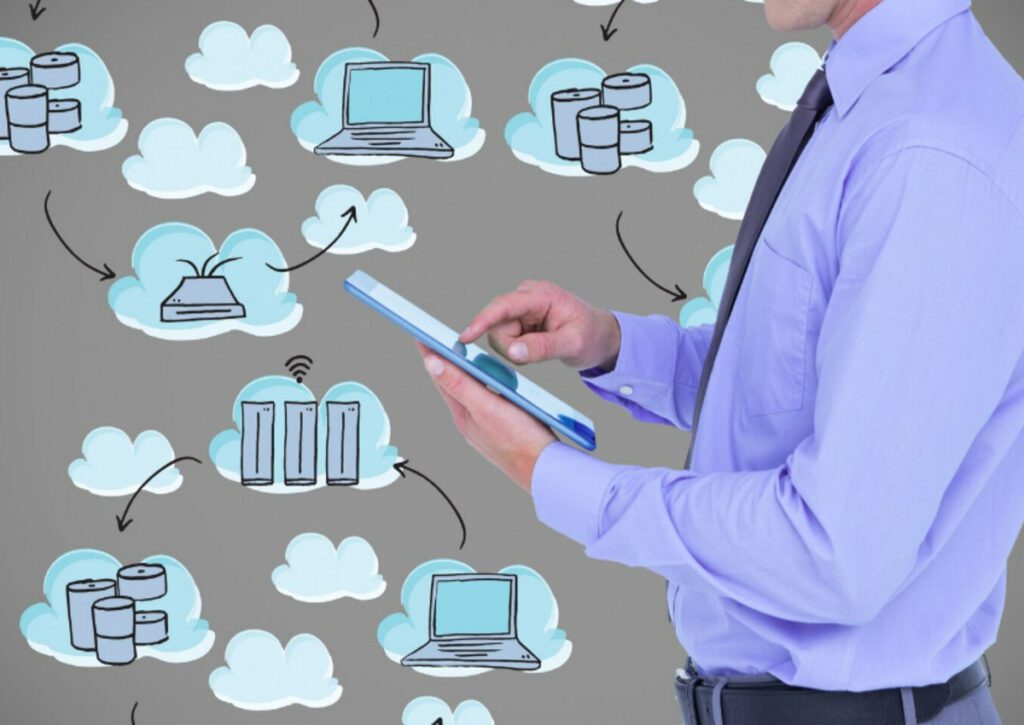
Implementing Machine Learning for Multi-Cloud Optimization
To effectively implement machine learning for multi-cloud network optimization, businesses should follow these steps:
- Data Collection and Integration: Gather and integrate data from all cloud platforms to provide a comprehensive view of the network. This data is crucial for training machine learning models.
- Model Training and Validation: Develop and train machine learning models using historical and real-time data. Validate these models to ensure their accuracy and reliability.
- Deployment and Monitoring: Deploy the machine learning models into the multi-cloud environment. Continuously monitor their performance and make adjustments as needed to maintain optimal results.
- Continuous Improvement: Machine learning models should be regularly updated and refined based on new data and evolving network conditions. This ensures that they remain effective in optimizing the multi-cloud network.
The Future of Machine Learning in Multi-Cloud Optimization
As technology advances, the role of machine learning in multi-cloud network optimization is expected to grow even more significant. Future developments may include:
- Advanced AI Integration: Combining machine learning with artificial intelligence (AI) could lead to more sophisticated and autonomous network management systems capable of self-optimization and self-healing.
- Enhanced Predictive Capabilities: Improved predictive models could provide even more accurate forecasts, allowing businesses to preemptively address issues and optimize resources with greater precision.
- Broader Adoption of Edge Computing: The rise of edge computing will likely see machine learning algorithms being deployed closer to data sources, reducing latency and improving real-time decision-making capabilities.
- Increased Focus on Security: As cyber threats become more sophisticated, machine learning will play an increasingly vital role in enhancing security measures across multi-cloud environments, offering real-time threat detection and response.
Conclusion
Machine learning is revolutionizing the management and optimization of multi-cloud networks. By leveraging predictive analytics, automated resource allocation, enhanced security measures, and optimized traffic management, machine learning empowers businesses to achieve superior performance and cost efficiency. A multi-cloud ML strategy offers significant advantages, including vendor flexibility, system resilience, scalability, and access to cutting-edge innovations. Despite challenges such as complex management and security concerns, the benefits far outweigh the drawbacks. As technology advances, machine learning will become increasingly integral to multi-cloud environments, driving more sophisticated, secure, and efficient cloud management. By adopting machine learning for multi-cloud optimization, businesses can ensure robust performance, strategic growth, and a competitive edge in the digital landscape.
FAQs
1. What is a multi-cloud network?
A multi-cloud network uses multiple cloud services from different providers to meet various business needs, enhancing flexibility, scalability, and data security.
2. How does machine learning improve multi-cloud network security?
Machine learning enhances security by detecting anomalies and potential threats in real-time, enabling rapid responses to mitigate risks and protect sensitive data.
3. What are the advantages of a multi-cloud ML strategy?
A multi-cloud ML strategy offers vendor flexibility, resilience, scalability, and access to the latest innovations, helping businesses stay competitive and adaptable.
4. What challenges are associated with implementing a multi-cloud ML strategy?
Challenges include complex management, integration issues, security concerns, and cost management, all of which require advanced tools and skilled personnel.
5. How can businesses implement machine learning for multi-cloud optimization?
Businesses should gather and integrate data from all cloud platforms, train and validate ML models, deploy and monitor them, and continuously update and refine the models to maintain optimal performance.